All About ML: Exploring the World of Machine Learning
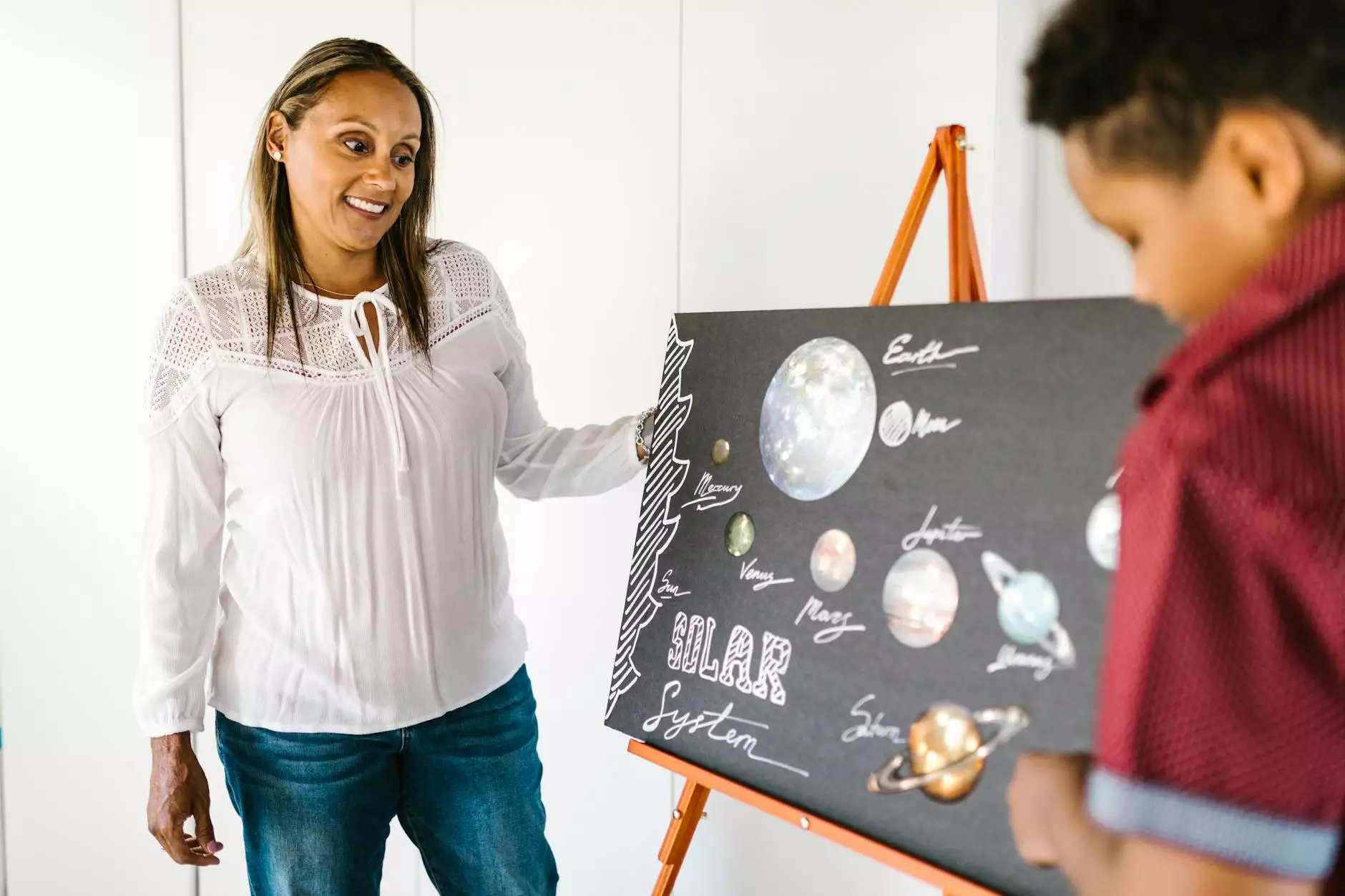
Machine Learning (ML) has become an essential facet of modern technology and business operations. Understanding all about ML opens doors to numerous opportunities in various industries. This article aims to give you an in-depth look at ML, discussing its principles, applications, and the transformations it brings to the business landscape.
What is Machine Learning?
Machine Learning is a subset of artificial intelligence (AI) that allows computers to learn from and make predictions or decisions based on data. Instead of being explicitly programmed to perform specific tasks, ML algorithms use statistical techniques to analyze and interpret patterns within data. This ability to learn and improve over time without human intervention is what makes ML a revolutionary technology.
Key Concepts of Machine Learning
To fully grasp all about ML, it's crucial to understand some key concepts:
- Supervised Learning: In this approach, the model is trained using labeled data. It learns the relationship between input features and the output to make predictions.
- Unsupervised Learning: Here, the model is provided with input data without labeled responses. It attempts to find patterns or groupings in the data on its own.
- Reinforcement Learning: This type of learning involves training an agent to make sequences of decisions by rewarding it for good actions and penalizing it for bad ones.
- Neural Networks: Inspired by the human brain, these are algorithms designed to recognize patterns. They consist of interconnected nodes, or neurons, that process data in layers.
- Deep Learning: A subset of neural networks with multiple layers (deep architectures), allowing the model to learn representations at various levels of abstraction.
The Importance of Machine Learning in Business
As businesses become more data-driven, understanding all about ML is vital for maintaining a competitive edge. Here are some of the ways ML is transforming businesses:
1. Enhanced Decision Making
ML enables businesses to make decisions based on data-driven insights rather than intuition. By analyzing historical data, businesses can identify trends, forecast outcomes, and make informed choices.
2. Automation of Routine Tasks
Many businesses automate repetitive tasks using ML, freeing up human resources for more strategic activities. This shift can significantly increase efficiency and reduce operational costs.
3. Improved Customer Experiences
With tools like recommendation systems, companies can personalize customer interactions and product offerings. This not only enhances customer satisfaction but also drives sales.
4. Predictive Analytics
Businesses employ ML algorithms to predict customer behavior, sales trends, and market movements. This foresight helps in better inventory management, marketing strategies, and resource allocation.
5. Fraud Detection
Financial institutions heavily rely on ML for detecting anomalies and fraudulent activities. By learning from historical data, ML models can flag suspicious transactions in real-time.
Applications of Machine Learning Across Industries
All about ML also involves recognizing its versatile applications across various sectors. Here are some noteworthy examples:
1. Healthcare
In healthcare, ML is revolutionizing diagnostics, treatment planning, and patient care. Algorithms analyze vast amounts of medical data to identify disease patterns and support clinical decision-making.
2. Retail
Retailers utilize ML for inventory management, demand forecasting, and enhancing customer experiences through personalized recommendations, leading to increased sales and customer loyalty.
3. Finance
Financial institutions apply ML in risk assessment, credit scoring, and trading strategies, enabling them to respond quickly to market changes and improve customer service.
4. Manufacturing
In manufacturing, ML is leveraged for predictive maintenance, quality control, and supply chain optimization, reducing downtime and enhancing product quality.
Challenges and Considerations in Machine Learning
Despite its many advantages, there are challenges associated with implementing ML:
- Data Quality: The effectiveness of ML models relies heavily on the quality of data. Poor data can lead to inaccurate outcomes.
- Bias in Models: ML algorithms can unintentionally learn biases present in the training data, leading to unfair or discriminatory outcomes.
- Interpretability: Many complex models, especially deep learning ones, act as "black boxes," making it difficult for businesses to understand their decision-making process.
- Security Risks: ML systems can be vulnerable to attacks, such as adversarial attacks, which attempt to deceive models by introducing misleading data.
The Future of Machine Learning
The future of all about ML looks promising, with continuous advancements in technology and methodology. Key trends shaping its future include:
- Integration with IoT: The rise of the Internet of Things (IoT) will provide vast amounts of data for ML algorithms to analyze and act upon, creating smarter environments.
- Explainable AI: As ML becomes more entrenched in business, the demand for interpretability will grow, fostering the development of models that explain their reasoning.
- Automated Machine Learning (AutoML): Tools are emerging that automate the selection and tuning of ML models, enabling non-experts to create effective ML solutions.
- Ethical AI: Addressing the ethical implications and biases in ML will become a primary focus, leading to development frameworks that prioritize fairness and accountability.
Conclusion
Understanding all about ML is more important than ever as businesses harness the power of technology to drive success. Whether it is through enhancing decision-making capabilities, automating processes, or improving customer experiences, the implications of ML are vast and profound. Embracing these changes will not only support a company's growth but also foster innovation and efficiency across industries.
To stay ahead in this rapidly evolving landscape, businesses must continually adapt and explore the latest advancements in machine learning. With commitment and the right strategies, the transformative potential of ML can be fully realized.