The Ultimate Guide to Labeled Image Datasets
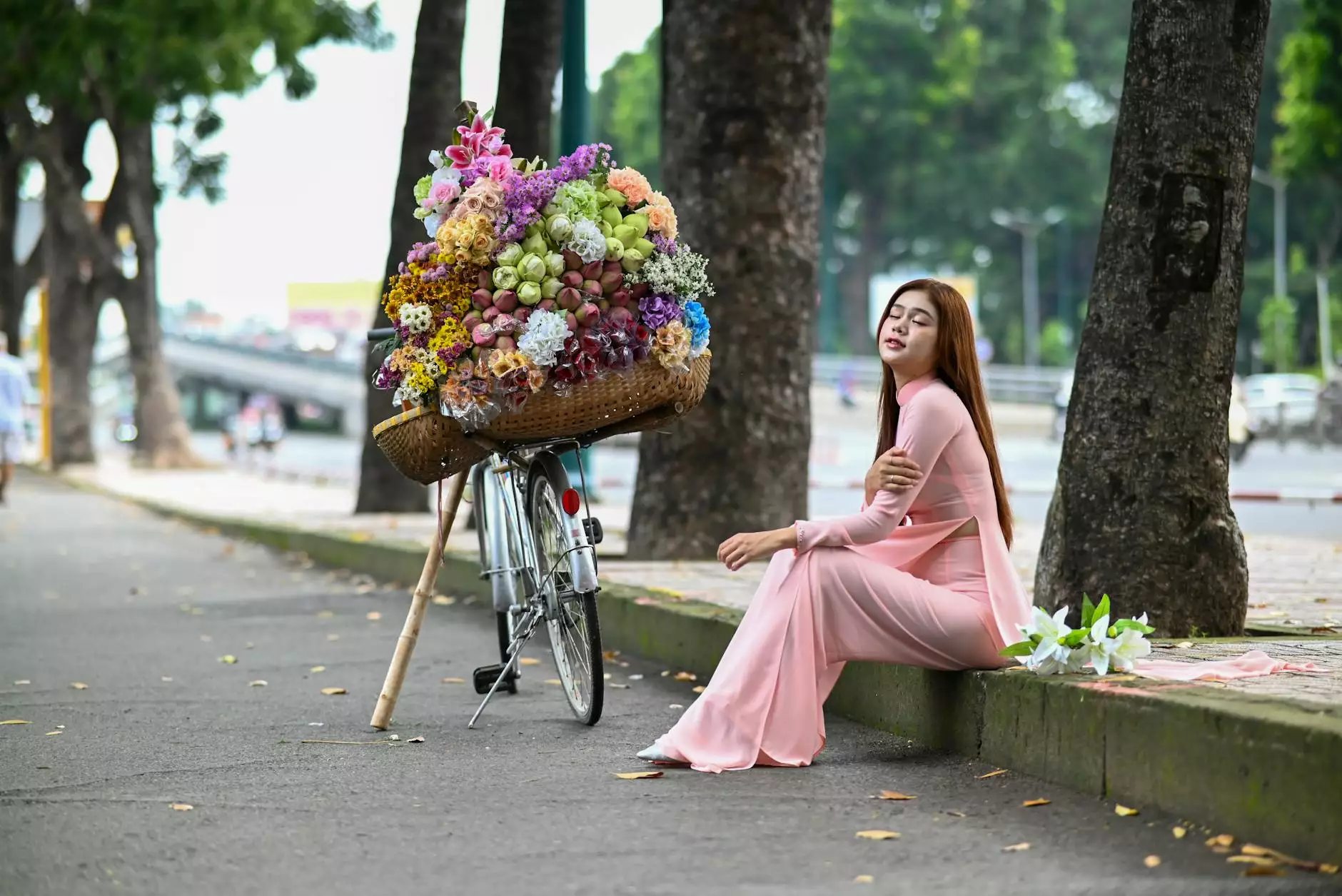
The world of artificial intelligence (AI) and machine learning (ML) is rapidly evolving, with labeled image datasets playing a crucial role in enhancing the performance of various algorithms. A well-curated dataset can significantly improve model accuracy, enabling systems to make informed predictions and decisions. This article delves deep into the concept of labeled image datasets, their importance, the process of data annotation, and how KeyLabs.ai is at the forefront of this technological evolution.
What Are Labeled Image Datasets?
Labeled image datasets are collections of images that have been annotated with descriptive labels. These labels provide essential information about the contents of each image, which can include objects, attributes, or even contextual information. For example, in a dataset designed to recognize vehicles, images may be labeled as "car," "truck," or "motorcycle" based on what is depicted.
These datasets are foundational for training machine learning models, particularly in the field of computer vision. By using labeled data, algorithms learn to identify patterns, make predictions, and ultimately achieve desired outcomes without direct human intervention.
Importance of Labeled Image Datasets in Machine Learning
The significance of labeled image datasets cannot be overstated. Here are some key reasons why they are vital for machine learning:
- Data Quality Determines Model Success: The accuracy of a machine learning model is heavily reliant on the quality of data it is trained on. Labeled datasets provide a structured approach to feeding relevant information into algorithms.
- Facilitates Supervised Learning: Labeled datasets are essential for supervised learning, allowing models to learn from a specific set of examples.
- Improves Accuracy and Reliability: With quality labeled datasets, models can achieve higher accuracy in tasks such as image recognition, object detection, and more.
- Enables Automation: Accurate labeled data leads to better automation in processes such as image categorization and analysis.
The Process of Creating Labeled Image Datasets
Creating a labeled image dataset involves several steps:
Step 1: Data Collection
The first step is to gather images from various sources. This could include photographs, screenshots, or images sourced from public databases. The more diverse the collection, the better the model can understand different scenarios.
Step 2: Data Annotation
Data annotation is the process of labeling images. This can be done manually or through automated tools. Manual annotation typically involves human annotators who tag images according to predefined guidelines, while automated tools can use algorithms to make predictions based on existing models.
Step 3: Quality Assurance
After the initial labeling, it is critical to conduct quality assurance checks. High-quality datasets rely on the accuracy and consistency of the labels assigned. This often involves reviewing a sample of annotated images and correcting any discrepancies.
Step 4: Data Formatting and Storage
Once annotated, the dataset must be properly formatted and stored for easy access and management. This could involve converting the dataset into a specific file format and ensuring it is organized in a user-friendly way.
Benefits of Using KeyLabs.ai for Data Annotation
KeyLabs.ai specializes in providing top-tier data annotation tools and platforms. Here’s how they enhance the quality of labeled image datasets:
- Advanced Annotation Tools: KeyLabs.ai offers a range of intuitive tools that make the annotation process efficient and accurate.
- Scalability: Whether you need a small dataset or a large one, KeyLabs.ai can scale their services to meet your requirements.
- Expert Research Team: With a team of specialists, KeyLabs.ai ensures that your datasets are annotated with an expert touch, leading to higher quality outputs.
- Quality Control Mechanisms: Multiple layers of quality assurance ensure that every label is accurate and reliable.
Applications of Labeled Image Datasets
Labeled image datasets are employed across various industries. Some notable applications include:
1. Medical Imaging
In healthcare, labeled datasets are critical for training models to detect diseases from medical images. For instance, annotating images of x-rays or MRIs can help in diagnosing conditions such as pneumonia or tumors.
2. Autonomous Vehicles
Self-driving cars rely heavily on labeled datasets to understand traffic signs, pedestrians, and road conditions. These datasets must be extensive and diverse to cover a wide array of driving scenarios.
3. Security and Surveillance
Labeled image datasets enable security systems to recognize faces and identify potential threats in real time, thereby enhancing safety measures.
4. Retail and E-commerce
In retail, these datasets facilitate visual search capabilities, allowing consumers to find products by uploading images. Accurate labeling is essential for effective retrieval of relevant items.
Challenges in Creating Labeled Image Datasets
Despite the importance of labeled image datasets, several challenges can arise during their creation:
- Time-Consuming Process: Manual annotation is labor-intensive and can require significant time investments, especially for large datasets.
- Consistency Issues: Maintaining consistent labeling across different annotators can be challenging, which can lead to discrepancies.
- Domain-Specific Knowledge: Certain fields may require annotators to possess specialized knowledge, making it difficult to find suitable personnel.
- Bias in Datasets: If the dataset is not diverse, it could lead to biased models that do not generalize well to real-world scenarios.
The Future of Labeled Image Datasets
The future of labeled image datasets is bright, driven by continuous advancements in technology and growing data needs. Here are some trends to watch:
1. Automation in Data Annotation
AI and machine learning tools are becoming more sophisticated, enabling automated annotation processes that can dramatically reduce time and labor costs.
2. Synthetic Data Generation
As AI continues to evolve, the generation of synthetic data—data that is artificially created rather than obtained from the real world—will become increasingly viable for training models, thus complementing labeled datasets.
3. Open Data Initiatives
With a growing recognition of the value of shared data, more organizations are participating in open data initiatives, making high-quality labeled datasets publicly available for research and development.
Conclusion
In summary, labeled image datasets are a cornerstone of modern machine learning applications, providing the necessary information for algorithms to learn and function effectively. The process of creating these datasets is pivotal for the success of any AI initiative, and platforms like KeyLabs.ai are leading the charge in quality data annotation.
As businesses continue to understand the importance of accurate and well-annotated data, investing in the right tools and services becomes essential. By leveraging the expertise and technology at KeyLabs.ai, companies can transform their labeled image datasets into powerful assets that drive innovation and growth.